One important conclusion from our previous analysis on artificial intelligence business is that technology can help businesses to capture, create and deliver value but that this is only possible as long as the technology is fit for purpose, i.e., can deliver tangible benefits to businesses once it is embedded in the architecture of a business model. Of course, this implies that it is necessary to take a closer look at the technology itself but more importantly, at the characteristics of the technology industry. This is not surprising: (very) large tech companies lead the effort to develop artificial intelligence business capabilities. In this respect, whether the technology is fit for purpose is very much a result of how the tech companies decide to compete in this arena.
As mentioned above, a critical development in the AI industry is the growth of AI-as-a-service where AI capabilities are offered as part of platforms such as Watson or Google Machine Learning. [supervised machine learning, unsupervised machine learning] Large tech companies have established the “platform” model which relies heavily on network effects to grow as a very successful mechanism to create and capture value: ultimately the whole digital economy relies on platforms and the associated eco-systems of users to deliver and capture value. Platforms allow to gather information on users efficiently and allow to create markets where services and products are exchanged. In turn, platforms facilitate the creation of platform- based businesses whose primary purpose is to facilitate transactions between other businesses and consumers and help generate eco-systems of interdependent businesses [Machine Learning Introduction].
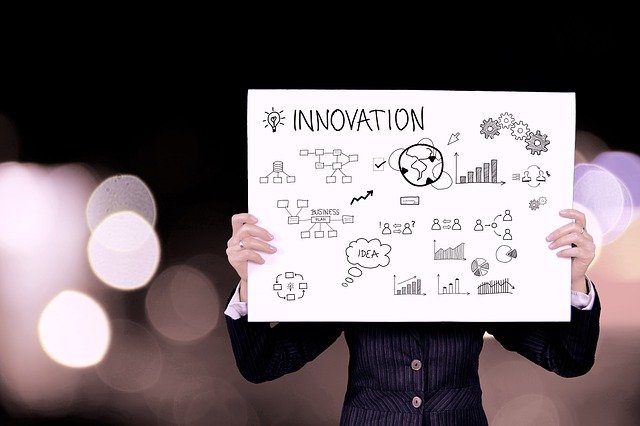 |
Artificial Intelligence Business Model |
While some authors argue that platforms are different from eco-systems in reality, platforms are needed to develop ecosystems. They both rely on interactions between the digital medium and many users, and in reality, most of the value created by platforms is in the number of interactions. Some interactions are bidirectional (where more organisations are part of the interaction) while some other interactions are not. In both cases, companies interact with users and extract value from these interactions outside their boundaries. The platforms deliver consistent components, define common interfaces as well as the technical standards. Therefore, they create opportunities for businesses and developers as they offer AI-related services at a fraction of the cost.
As the platform provider becomes the orchestrator of the eco-system (or of the network), platforms provide an environment where software is integrated, and access to training data is guaranteed well as labelling services and consulting. In other words, platform providers tend to offer an environment where products can be standardised, although still differentiated from those of the competitors. In this area, artificial intelligence business services allow platform providers to provide a set of highly specialised services that are cheap to maintain given access to training data and hardware [what is big data?].
What are the implications of artificial intelligence business on the market structure?
In the case of platforms, a platform owner’s main objective is to maximise participants on the two sides of the market. As a result, the platform owner will always increase users’ number (whether paying or not) of its platform. In the current environment where platforms provide artificial intelligence business services, switching costs can be very high as the availability (or lack of) training data may make a business less likely to switch to other platform providers.
Availability of large training datasets is quite essential to businesses that want to use artificial intelligence business systems. Search engines embedded in platforms collect data on their users’ behaviour, which can be used as training data for AI. Thus, for other firms to benefit from AI, they need access to platforms with a large user base and the data that come with it. Access to training data may act as an entry barrier for new competing networks at the industry level. A related concern is an impact that concentration of data among a few dominant platforms may have on the artificial intelligence business system’s quality and even their use [impact of ai in business].
High switching costs and the nature of competition of technology markets narrow down the options that incumbent firms have in business model innovation. These issues may not be relevant to firms that have been built on platforms since the very beginning. Such business examples include Uber and Airbnb, which are platform businesses, and their main challenge was about gaining legitimacy in the eyes of customers. However, in the case of incumbents, this may be an issue as mature businesses may have to decide what mechanisms for value creation, delivery and capture they may want to adopt given that their access to artificial intelligence business services is controlled by large tech companies. The literature has pointed out that in practice platforms induce businesses to privilege business models characterised by openness. Business models that use AI at their core require businesses to access artificial intelligence business services through platforms and interact with many organisations that use the platform. Therefore, businesses have to learn to manage a much larger number of interactions across the whole value chain, i.e., not only with suppliers and customers. For instance, in product development, interactions with users through open innovation and innovation contests become common. Equally, the quality control function may be outsourced as businesses may rely on platforms to collect data on the quality of their products and services. External focus requires businesses to develop additional capabilities that help manage these new types of relationships and interactions with organisations that cannot be traditionally considered to be stakeholders.
Openness means that the business has to accept inputs from outside the traditional boundaries of the business. Examples include open innovation where innovation is developed collaboratively with inputs from across many external organisations. The shift towards openness requires a change in culture and internal processes so that externally sourced inputs can be successfully integrated into the new business model.
Welfare considerations. To conclude this subsection, we want to offer some reflections on the extent to which the nature of competition in technology markets is welfare-enhancing. It can be argued that the platform model adopted by tech firms may foster competition (at least among users) by inducing businesses to adopt business models characterised by openness. Empirically, we do not have any evidence on the magnitude of these effects. A related issue is whether the platform model can enhance innovation among tech firms. Existing work on competition and innovation points to the existence of two counteracting effects: on the one hand, more intense product market competition (or imitation threat) induces firms at the technological frontier to innovate in order to escape competition; on the other hand, intense competition tends to discourage firms behind the current technology frontier to innovate. Which of these two effects dominates, in turn, depends upon the sector. The implications for the development of AI may be interesting. As entry costs into the technology markets become larger and larger (as the availability of training data acts as a barrier to entry), it is unclear whether companies that invest to be closer to the frontier may be interested in continuing to do so as it may be more profitable to work on AI applications rather than on theoretical developments in AI.
Academic research tends to assume that radical innovation (like AI) will lead automatically to improved business performance (once the new technology is embedded in the existing business model). Therefore it tends to ignore the interdependencies between business model choice and technology. However, technology has to operate with other technologies and therefore, it may create value if they work well. Interoperability is essential in particular if we consider platform technologies as they offer opportunities for complementarities, which can enhance the value capture mechanisms. Interoperability is linked to technical standards, and in this subsection, we will focus on technical standards and how they support new business models that use AI.
Why Do We Need Technical Standards and What is Their Impact?
Standards can be of several types. Product standards can define measurements, requirements, labels and testing methods. Management process standards can describe processes to achieve goals such as quality3 or functional safety (processes to assess risks in operations and reduce them to tolerable thresholds). Network-product standards that support interoperability and network-process standards are used to grow their market size and reduce their costs.
Most of the literature on standards has focused on industry players’ rationale to introduce standards and their impact. At a fundamental level, standards are introduced to reduce asymmetric information between buyers and sellers. Asymmetric information is common to most markets, and it arises every time the seller has more information about the quality of a product than consumers. To understand how standards work, consider the incentives of economic agents and the objective of standardisation process: in a world, characterised by uncertainty about the quality of the products, standards can make explicit the production process and clarify to users the specifications of the products as well as the processes followed for their production.
A few studies have tried to clarify the impact of standardisation on innovation. One way to formalise the impact of standards on innovation is by considering standards as a mechanism that reduces the current and future transactions costs. Besides, standardisation is a frame- work within which future standards can be produced. In this respect, it limits the variety of options and induces firms to develop credible technologies in the eyes of the consumers and can support the development of complementary technologies. From the innovator’s standpoint, the presence of standards in the market justifies the investment to produce the new products at scale. This way, businesses can generate profits which can let them reap the benefits of the initial investments. Importantly, standards may create trust in new products, leading to acceptance among consumers by making it clear to consumers how risks have been mitigated. Although some economists have suggested that standards may slow down radical innovation, standards can avoid these lock-in effects and compatibility over time is ensured. Additional benefits of standardisation for innovative firms include:
- Development of a critical mass of innovators in emerging industries. Standardisation may create a critical mass of innovators in emerging industries and promote innovative products . In particular, standards allow the development of complementary innovations.
- Diffusion of technical information. Standards clarify that innovation has the features that producers claim are there and that it is safe to use it.
- Diffusion of best practice in industries. Standards help firms diffuse best practice in manufacturing and technology while allowing first movers to gain some benefit from licencing standards. Besides, standards can set the minimum requirements for environmental, health and safety impact of new products.
- Increase competition in an industry. This effect works in two ways. On the one hand, standards can generate competition among technologies that can benefit the economy as a whole. On the other hand, technical standards can level the playing field among businesses in the industry.
Technical Standards and artificial intelligence business
There are two areas related to AI associated with the development of technical standards. The first area is around AI safety, and the second one is around artificial intelligence business systems capabilities. The field of AI safety is young, but given its potential impact on the future developments of the technology and its industrial uses, standards need to emerge relatively quickly. One of the critical issues in implementing safety processes at scale and this issue needs to be developed into research on technical safety itself. A starting point would be the existing safety standards for emerging technologies as developed by international standards bodies. The best way forward is to develop the current best practice and develop a set of processes enshrined in a standard that helps researchers develop a checklist before undertaking research. The process standards could contain the exact specification of the code and validation methods. Finally, the best practice could establish how to monitor standards and define the thresholds above which risk can be considered so high that different procedures need to be taken into account.
The arguments in favour of their introduction are well-rehearsed:
- Communication among researchers and policy-makers. The development of technical standards can facilitate communication among the number of institutions and bodies working in the field. This is a notion that underpins the concept of standards according to several authors. Indeed, both Swann (2000, 2010) and Blind (2006) suggest that standards are devices that codify organisations’ tacit knowledge. Codification is useful for the diffusion of the technology which relies on exchanging what could be otherwise tacit knowledge. In the case of AI, standards may facilitate communications, facilitating the development of trust. In this respect, standards may act as a mechanism to retain the benefits of private investment in the new technology mitigated by public intervention benefits. The standardisation process can facilitate the diffusion of technical information.
- Coordination. Technical standards are a crucial mechanism to coordinate producers’ activities along the supply chain and ensure interoperability among the several components. Standards elaboration allows industry players to select relevant knowledge and technologies and avoids industry fragmentation. Blind and Gauch (2009) suggest that standards are a channel for knowledge transfer through a consensual process. This way, R&D results can become public goods through standards that are accessible to everybody and are broadly implemented because all industry players have reached a consensus on their content (Farrell and Simcoe, 2012).
- Time to market and future developments. Standards offer several opportunities to the AI’s growth by reducing the time to market for inventions and technologies. Using the arguments developed by Blind et al. (2011), there are four main channels through which standards enhance the development of Artificial Intelligence:
- Standards can minimise coordination costs. These can be important for the development of technologies that work as platforms to host apps.
- Standards allow firms to exploit economies of scale.
- Standards can increase the demand for complementary products and services that can be routed through the AI-powered platforms.
Standards provide the institutional framework that allows companies to develop new technologies in a controlled and safe manner, which is very important in the context of AI, which can be deployed across several industries and several applications.
The approach to the growth of an industry around new technology is based on public funding of R&D and IP rights. The assumption is that increases in publicly funded R&D and a robust IP regime may facilitate the private sector investment in AI. In the context of AI, however, it is questionable whether this is the case. Indeed, the development of AI technologies is a very diffuse process that involves many actors, which makes it necessary to develop effective mechanisms for fast technology transfer. In this sense, standardisation can be such a mechanism and help the process of knowledge diffusion, which underpins the AI industry’s development. Also, in the context of AI development, users are an important actor within the innovation process and standards can be used to coordinate the activities of several actors and stimulate future research in AI. Additional standards may be used to shape the R&D process, emphasising safety and the development of an ethical framework. Standards may provide information about other businesses which may lead to the development of other standards in the future and to identify the most efficient technology that may lead to the development of advanced artificial intelligence business systems.
Interoperability and international cooperation. Standards promote outsourcing of specific tasks to more efficient producers. For example, it may be optimal for a company to contract a supplier with lower input costs to manufacture their products while they focus on the design of the products. Simultaneously, by improving compatibility between components, producers can adapt products or processes according to the demand quickly. These standards may help support the growth of AI-based on systems that are implemented using consistent processes. In other words, standards may facilitate AI technology deployment, which will increase the global market for artificial intelligence business systems. Development of AI has quickly become a global challenge as governments worldwide have started to support AI research within their countries but with very little attention to the global landscape. There is a risk this may lead to a fragmented governance landscape and a race to the bottom in terms of the regulation. Therefore, AI standards have to be international. Indeed, international standards have a history of guiding the development and deployment of new technologies that significantly impact society.
The main challenge that decision-makers face in AI standards development is how they can hinder innovation in the field. There is a long-standing view as they can limit their capability to extract a return from their initial investment in innovation. While it is unclear the extent to which this hypothesis is confirmed by empirical analysis, it is still an argument that underpins debates on standards in the context of AI development. Therefore, we must examine the mechanisms through which standards may impact AI innovation and eventually identify conditions under which the introduction of standards can result in an AI development slowdown.
Benefits of Standards for Business Model Innovation
As for the impact of technical standards on business model innovation, there is hardly any research. It could be argued that standardisation is simply a time-consuming process which produces minimal benefits to firms. It has been argued that incentives to join standardisation processes are limited because of opportunity costs as these efforts limit the competitive advantage that lack of standards offers.
While there is some debate on these arguments, standards are beneficial for early technologies that can change cur- rent business models. Significantly, compatibility standards can promote the diffusion of technologies and products in network industries. In these cases when there are emerging technology fields, standards may create the conditions to set flexible framework conditions that can be transferred into new business models that can be developed further when the technology is mature. Based on the previous discussion on the three main components of a business model (components that are arranged around the core mechanisms for value creation, value delivery and value capture), we can argue that the development of technical standards for AI can shape the design of mechanisms for value creation and value capture.
As for value creation, standards reduce costs associated with the adoption of AI and the costs of developing further AI-based applications that can solve business-specific issues. As a result, standards can incentivise businesses to adopt new business models that privilege value creation through cost reduction. As for value capture, standards allow businesses to capture value by developing new products that are triggered by the compatibility of the different components of AI. This is a departure from the traditional value capture model where protection of intellectual property and price structure are the standard mechanisms to capture value.
In this respect, an interesting issue here is about the relationship between patents and standards. One argument is that the integration between IP and standards can enhance AI innovation as it would provide businesses with more mechanisms to capture value. Combining the two activities creates incentives to invest in innovation and ensures that businesses invest resources in technologies that have significant potential in terms of diffusion. These patents can then be licensed by the patent holder using the Fair Reasonable and Non-Discriminatory (FRAND) condition although Blind et al. (2017) suggest that the accumulation of licencing fees by different owners may generate increasing licencing costs.
There are many counterarguments, in any case. First, patents give the holders some temporary monopoly that the integration can enhance into standards, which may last longer than patent protection. Second, it would make no sense to combine patents and standards in platforms as revenue is dependent on the indirect network effects generated by further innovations that rely on platform technologies. Third, it is essential to recall that standards are produced once a specific technological specification has been selected. Whether this is the best technology, it is unclear although Rysman and Simcoe (2008) have provided empirical evidence that standard-setting organisations select successfully patent protected technologies, which are superior to other available technologies. Finally, the integration between patents and standards may lead to conflict between the standards body and the patent holders. For instance, compliance with standards may infringe a patent which is not part of the standards.
The Institutional Framework: Regulation vs. Standards
One of the areas of discussion on AI is whether standards (voluntarily agreed) are a replacement for regulation. This is an essential topic in the context of AI given the industry structure, which spans several sectors and typologies of businesses. For
these reasons, we will discuss these arguments and discuss the extent to which regulation and standards can complement each other in the context of AI. In a nutshell, the literature suggests that to support AI development, regulatory bodies may be problematic while standards can provide rules that developers can trust.
Before starting the discussion on the relative merits of regulation and standards, it is worth recalling that regulation is a coercive rule- setting while standardisation is a self-regulatory activity. The impact of regulation on innovation has been discussed in academic literature. Complying with regulations can be costly for incumbents, and there- fore it may restrict their capability to innovate. Regulations are mandatory restrictions released and enforced by the government to shape the market environment and influence businesses’ behaviour. Correspondingly, regulations refer to a top-down approach, while formal standards are typically the result of a market-driven process.
Whether regulation has to be preferred to standardisation depends on the maturity of the technology. Indeed, Blind et al. (2017) have highlighted that technological complexity (like in the case of AI) generates uncertainty on the best practice that should be formalised in a formal standard. In such environment, setting standards according to technological preferences and potentially raising rivals’ costs is expected to be much difficult and standard-setting bodies may end up work around one particular standard which may not be the optimal one. Consequently, in highly uncertain technologies, regulation may be a better option than setting standards. When the technology is more mature, it is preferable to gain revenues by expanding the markets and ensuring interoperability.
Ethical Framework
This subsection will focus on the role that
ethical frameworks can play in constraining business model innovation. Typically, when organisations start to invest in AI, they tend to focus on the opportunities that the investment can bring, and most of the discussion is the costs and benefits that the opportunities may offer. However, minimal effort is given to how the new technology’s deployment is aligned to the current organisational thinking around ethics.
Ethics is usually thought of as a framework to mitigate the risks associated with AI’s widespread adoption. Crucially, some are direct and are linked to the direct use of AI-powered systems generate while others are indirect. Traditionally, these risks are dealt with frameworks which are very rooted in business ethics. However, this attempt has not been very successful for two main reasons: first, business ethics offers a framework to think about ethical issues in a business, but it does not provide criteria that can support decision-making . Second, business ethics is not equipped to deal with technologies – such as AI – that can make decisions in an autonomous way following rules that are not apparent to the users.
Companies are aware of these issues and have tried to embed ethical decision-making in the design. For example, IBM stress that managers should be put into the position to override decisions made by artificial intelligence business systems if desirable, and that bias reduction should be considered in the design of artificial intelligence business systems, too. The European Commission’s AI High-Level Expert Group stresses that AI must be “legal, ethical and robust”, i.e., it needs to prevent harm, especially for vulnerable people, and take into account the broader societal risks.
Several principles that guide organisations when dealing with AI and ethics:
- Both positive and negative impacts have to be considered.
- AI has to complement humans
- Humans need to be in control
- Human Safety and Wellbeing need to be preserved
- Decisions need by AI systems need to be consistent with human rights
- Decisions need to be transparent, and there has to be an audit trail.
- Processes for Quality Assurance need to be made explicit.
- AI systems need to be robust and resilient.
- The principle of accountability needs to be preserved.
- A legal framework around the use of AI systems needs to be put in place.
There are additional issues around the use of AI that need to be considered. AI requires data collection, and in this respect, the ethical issues are not very
different from other types of analytics. Of course, several ethical issues arise when dealing with data collected by AI systems. These data may be sensitive and personal. An example is provided by the data collected by AI-systems deployed in a healthcare context. By definition, they can be sensitive, and besides, patients may not be aware that the data are collected. Finally, the patient may feel it cannot opt-out in this situation [
What is data science].
For the ethical framework to support business model innovation, it is essential to go beyond the general principles established by groups of experts and by legislation and focus on AI’s actual position within the business model. As a minimum, this requires each company to establish a governance framework that will support the deployment of AI internally to support new ways of creating and capturing value. So far, governance has focused primarily on privacy protection with policies for handling sensitive personal data. Typically, this has been done in the context of the legislation on privacy protection. This cannot allow for exceptions based on the requirements of industries or even single companies. However, one fundamental limitation of the
legislative tool is that it cannot deal with change and transitions in a fundamentally dynamic and contextualised way. Their role is to “frame” decisions and situations and encapsulate patterns of behaviour; not to facilitate simple steps and activities that can take on a “unique turn” in any given situation or even present singular and unique questions for a particular case.
Some authors have suggested something similar, although in the context of ethical data collection and re-use. For instance, Richards and King (2013) suggested developing an organisational framework for data’s ethical use. Hoffmann et al. (2012) recommend establishing a small decision-making body made up of representatives of business leaders, user communities, data suppliers, and technical staff to give stakeholders some control over data use [
components of data science].
Beyond the use of data, the development of a framework for the ethical use of AI needs to understand AI applications’ specific context in a much more nuanced way. This is important if we hope to:
(a) consider the continually evolving nature of technology and its uses and (b) “break free” from the question of the primacy purported of either regulation or
standards that permeates discussions and decisions on AI’s possibilities as described above. This will require a new way to engage with and lead implementation processes. Such a premise requires two starting points. The first one is about shifting our gaze beyond a technology-driven view that, commonly, focuses strongly on either the adaptability of technology or the adaptive capabilities of actual people and stakeholders involved where humans are not just being seen as mere passive receivers of top-down decisions or “following” actions and instructions already developed elsewhere. Such a step will require to engage with a different understanding of change involved in AI applications’ design and implementation.
Such a framework requires understanding whereby people’s every- day practice can gain centre stage in the process and create trust, transparency, and accountability. We will use the main news story for elaborating on our approach. As of August 2020, one of the leading news in the UK was what large parts of the British public perceived as being a “scandal” or mismanagement in the use of
machine learning algorithms to assign the final high school grades due to the cancellation of exams in the year of the pandemic (2020). Apart from many other considerations, here, it is interesting that the “business model” adopted was seen by decision-makers as unquestionably the most appropriate way to guarantee fairness for all students. After the U-turn, the business model is still being seen as valid by those that chose it; however, it was at least accepted that problems happened in the process of “implementation”.
We purport that what “went wrong” was the excessive concern with regulation and privacy preservation underestimating the role of the process around the use of the algorithm and the need to engage key stakeholders such as teachers, students and others (including Universities) highlighting early potential problems and that was essentially “over-run” by AI.8 For the purpose here, this example shows a fundamental flaw in the governance model around the use of the AI in education, a flaw so fatal that a potential innovation in the model used to allocate grades has been dropped by the government because of the lack of trust between main actors and the top-down approach used to introduce it. The development of an organisational ethical framework that is flexible enough to facilitate business model innovation requires organisations to realise that AI arises in social space because of the different actors involved. In other words, AI cannot be managed, governed and sustained in any single place and thus is fundamentally distributed in nature:9 for instance, the development of
AI in a company is domi- nated by cross-functional teams, and each of them can be considered a stakeholder for the specific AI project; also, the impact of AI is well beyond organisational boundaries and may ripple through the local community to impact businesses, healthcare and overall well-being. This requires an alternative view of ethics to position AI, a view that identifies and recognises the participants well beyond the “usual” suspects. In this context, it is essential to be aware of the dynamics among the different stakeholders and how micro-politics can influence AI’s decision making and legitimise views of groups that held a position of power.
An interesting approach to developing an ethical framework for AI points towards collective ethics in the shared space of action. The implication is that decisions around the use of AI cannot be made by one person only but require several individuals’ contribution. In these cases, leadership is not centralised with one individual or a team, but it is distributed. There exist theories that explain why distributed leadership emerges and what benefits it offers. Distributed leadership can support the development of an ethical framework around AI while retaining sufficient flexibility for innovation. The use of this framework implies that ethics (and ethical frameworks) is defined as a collective social process emerging from the interaction of several actors. This approach highlights that members of a community have to both support and question the values and the uses around AI. Such an approach would allow companies to “suspend judgements”, thus avoiding hasty decisions and creating awareness about the increased need for a more inclusive space of action when using AI.
An example of a company that used AI to replace the existing scheduling methodology. The existing procedure was essentially manual and based on workers’
preferences and well- known conflicts of schedules. AI changed how the schedule was decided, but importantly the company allowed the planner to use their knowledge and expertise to make the final decision on the schedule. Crucially, the final decisions were not subject to managerial approval, and effectively it created a space where planners were allowed to make decisions and show their leadership in the matter. As a result, all planners adopted the tool as they felt the tool was helping them and supporting their decision-making.
Over the last five years, advances in AI technology have rekindled the academic interest into AI and its potential impact on organisations and society. While some of the academic discussion on AI tends to revolve around the technological advances, some researchers have tried to articulate the actual impact that AI can have on businesses’ core competencies, and performance as a research agenda distinct from the hype surrounds the technology itself.
Summarising this nascent literature was the objective of our monologue. First, we have described the relationship between AI and business model innovation and then discussed AI’s impact (as an emerging technology) on business model innovation. To this purpose, we have referred to the strategy literature that describes a business model as a set of connections among the mechanisms for value creation, delivery and capture. In other words, it describes how a business needs to be organised to create value that gets delivered to customers.
More specifically, we have used the literature on business model innovation to provide a framework to explain how businesses adapt and renew their business model once AI diffuses across industries. The framework itself has used elements of organisational learning theory; prior research suggests that the process of business model innovation is a learning process [
reinforcement learning], and therefore they build a theoretical framework to research into business model innovation. This
framework enables us to understand better and analyse how businesses rebuild their business models in a new setting; also, it shows how businesses learn about the possibilities the new technology offers and how the perception of these opportunities shapes the choices businesses make concerning the new mechanisms for value creation, delivery and capture.
We have also pointed out that experimenting is an essential aspect of embedding AI technologies into a new business model. In other words, the process of identifying new business models requires businesses to experiment with alternative ways of changing the way businesses can generate value and can do so as long as they can learn and identify what works given the constraints the business faces. Once these learning capabilities are in place, businesses can exploit the opportunities that AI offers to businesses very quickly. We find that businesses may follow the four patterns of business model innovations (such as changing the internal processes, improving customer interfaces, joining eco-systems and developing smart products), each varying in how they use AI to deliver, capture or generate value.
Finally, the monologue has tried to identify the industry-level factors that drive businesses’ preference for a specific business model. First, we have analysed how the technology industry structure – dominated by large tech firms that own technology platforms offering services to both consumers and developers induces businesses to prefer business models characterised by openness. Second, we have discussed how the introduction of technical standards acts as a tool to enhance AI adoption. AI standards development is already underway at both ISO/IEC and IEEE as they can support the diffusion of the technology. The claim here is that standards can produce expertise that may allow the industry to move towards a business model that moves away from protecting intellectual property and product differentiation as a source of revenue streams. In other words, in the presence of standards, businesses tend to choose business models characterised by alternative mechanisms for value capture and value creation that privilege volume rather than differentiation. Finally, we analyse the impact that alternative ethical frameworks may have on the preference for a specific business model.
Our analysis of the current literature on business models and AI suggests there are several gaps in our understanding of how businesses manage the challenges that the diffusion of AI generates. Although AI has become a technology of interest for most businesses, the extent to which these businesses struggle when trying to adapt their business models to the new technology is unclear. It can be argued that businesses that have been established for a while may find it difficult to accept the notion that their business model has to change, but in reality, we have no
data science workflow that support this somehow educated guess. There is, therefore, a need for more research on what prevents firms from changing their business model and how they can overcome these obstacles.
Second, it may be optimal to change the business model in some cases, but still, it is not clear who can be the agent of change. Our discussion on business models suggests that managers need to be creative when dealing with the interplay between AI and new business models. Importantly these discussions require an understanding of who can facilitate change. Importantly, different groups and teams can have different perspectives on how to trigger business model innovation. For instance, technologists may understand the possibility of the technology but may miss the implications for value capture; vice versa marketing executives may not have a technology insight. In this respect, a new class of experts that translates the benefits of analytics to marketing realms may be needed. Notably, while it is in the interest of a business to respond to the challenges posed by new technology, fostering a culture of innovation may be difficult in companies that have been established for long. In this case, it is up to the senior management to establish a culture that facilitates learning and innovation. Still, we do not have formal studies that confirm the extent to which senior management can play this role and whether other teams within the business have to support the senior management team’s activities. However, this issue has been analysed in a case-study presented by Fountaine et al. (2019) who reports of a bank that aligned its AI initiative to the existing organisational culture which may have acted as a barrier.1
Third, the current literature does not deal with the consequences of business model innovation. In other words, the current literature offers a snapshot of how businesses have changed the way they make business thanks to AI, but there is a small number of studies that tells us about the sustainability of these new business models and their dynamics over time. This is expected given the fact that AI is an emerging technology.
COMMENTS